Vector search is a method for finding similar entities (sentences, images, videos) using mathematical representations of these entities. These representations are called embeddings, which are expressed as vectors and then used to perform the vector search.
ℹ️ To find out more, check out our wiki on the basics of vector search : What is vector search?
The introduction of vector search in BigQuery
In January 2024, Google announced the integration of vector search in BigQuery as a preview through the function VECTOR_SEARCH,
and the CREATE VECTOR INDEX
which can be used to create hints to improve the search and calculation of distances between vectors.
Vector search in BigQuery requires a database containing embeddings to calculate distances between elements and assess their similarity.
There are several ways to create embeddings, however, we will focus on BigQuery's built-in functionality to do so: ML.GENERATE_EMBEDDING
.
The function ML.GENERATE_EMBEDDING
allows you to generate embeddings directly in BigQuery using a VERTEX AI model.
User guide :
In our detailed guide, we'll use vector search on BigQuery to find titles of similar articles in a database of bbc_news available to the public on the dataset bigquery-public-data
. The aim of this exercise is to find articles with a title similar to an article title of interest in the database.

Embedding generation :
As mentioned above, to perform a vector search, you need to use embeddings.
To create one, we detail the steps to follow:
Creating a dataset on BigQuery
This involves creating a new dataset on BigQuery to store the model we'll use to generate the embeddings, as well as the various tables we'll create later.
To do this, simply execute the following query:
CREATE SCHEMA `toolbox-starfox.vector_search_demo`
ℹ️ To find out more, visit BigQuery documentation.
Creating a connection to Cloud resources.
📖 To be able to create a BigQuery connection with Cloud resources, you need:
- Activate the BigQuery Connection API.
- Have the roles of :
- roles/bigquery.connectionAdmin
- resourcemanager.projects.setIamPolicy
- roles/bigquery.dataEditor
- roles/bigquery.user
- Create the connection with the Vertex AI model in BigQuery :

- Assign role
VERTEX AI user
to the connection service account :
Creating the template for embedding generation :
To create the model, simply execute this simple query:
CREATE OR REPLACE MODEL `vector_search_demo.embeddings_model_demo` REMOTE WITH CONNECTION `projects/toolbox-starfox/locations/us/connections/embeddings-test` OPTIONS (ENDPOINT = text-embedding-004);
ℹ️ There are several templates on Vertex AI for generating embeddings. To use the multilingual template, we usetextmbedding-gecko-multilingual@001
ortext-multilingual-embedding-002.
👉 For more details, please visit Google documentation.
📖 Don't forget to replace the dataset with the name of yourdataset
. and give yourmodel
.RENTAL
and
CONNECTION.ID
which you can find in the login details.
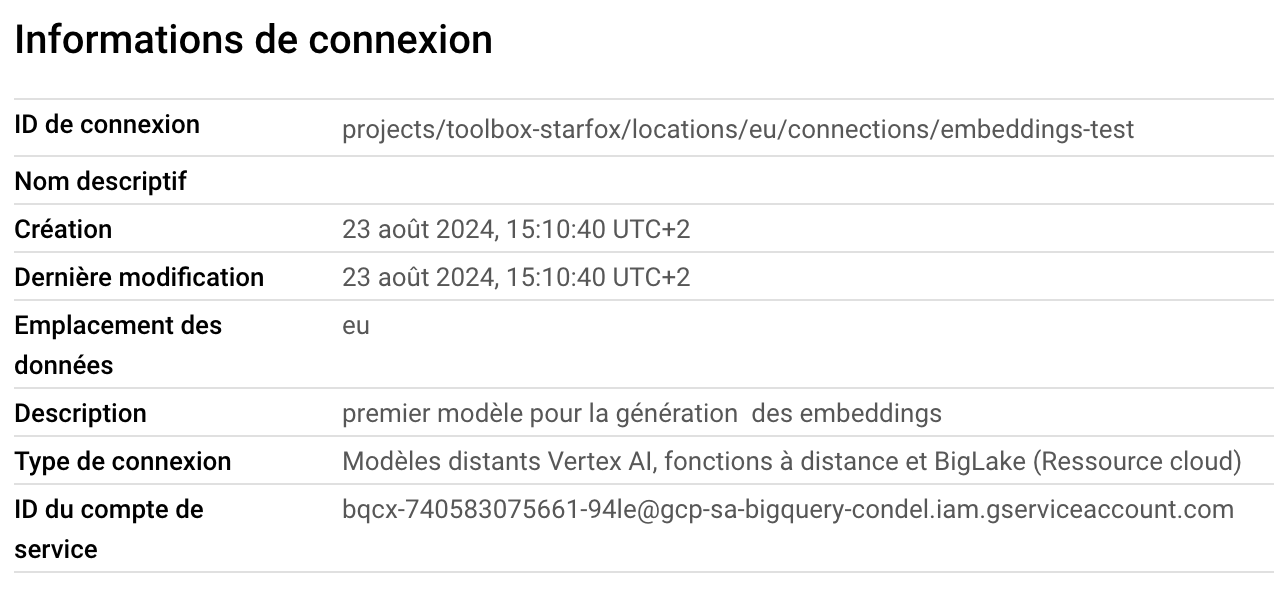
Embedding generation :
To generate the embeddings needed to apply the vector search, simply execute this query. In our example, we'll generate the embeddings for "title" from the bbc_news table.
CREATE OR REPLACE TABLE`toolbox-starfox.vector_search_demo.bbc_news_embeddings` AS (
SELECT *
FROM ML.GENERATE_EMBEDDING(
MODEL`toolbox-starfox.vector_search_demo.embeddings_model_demo`,
(
SELECT title AS content
FROM`toolbox-starfox.vector_search_demo.bbc_news`
),
STRUCT(
TRUE AS flatten_json_output,
SEMANTIC_SIMILARITY' AS task_type
)
)
);
ℹ️ For more details on this query's options, please consult the Google documentation.
Vector search index generation
A vector index is a specialized data structure that optimizes the function VECTOR_SEARCH
for faster searching among embeddings. Its principle is based on the ANN (Approximate Nearest Neighbor) technique.
The IVF method is a specific approach to creating a vector index. Here's how it works:
- Clustering with k-means
- Vector data are first grouped into clusters using the k-means algorithm.
- Each cluster represents a group of similar vectors.
- Partitioning
- The vector data is then organized according to these clusters.
- This creates an "inverted" structure where each cluster points to the vectors it contains.
- Optimized search
- When searching with
VECTOR_SEARCH
the algorithm can quickly identify relevant clusters.
- The search then focuses on these specific clusters, considerably reducing the search space.
- When searching with
This command creates a vector index on the specified column, using the IVF method and cosine distance to measure similarity between vectors.
CREATE OR REPLACE VECTOR INDEX my_index_bbc
ON `toolbox-starfox.vector_search_demo.bbc_news_embeddings` (ml_generate_embedding_result)
OPTIONS(
index_type = 'IVF',
distance_type = 'COSINE'
);
ℹ️ All elements of the embedding array must be noNULL
and all column values must have the same table dimensions. It is therefore mandatory to clean up your new table containing the embeddings (in our case: bbc_news_embeddings) by deleting the NULLs in theml_generate_embedding_result
. The table must contain at least 5000 for the CREATE VECTOR INDEX query with index type IVF. If this is the case, you can skip this step.
👉For more details on this query's options, see the Google documentation.
Using vector search
The last step consists in using vector search to find titles similar to a title in the table. The role of this query is to use the table we created earlier, which contains the embeddings, to search for titles with embeddings close to those of the title we're trying to analyze, which in our example is "PlayStation 3 chip to be unveiled", using the distance calculation method: COSINE .
SELECT
query.title,
base.content
FROM
VECTOR_SEARCH(
TABLE`toolbox-starfox.vector_search_demo.bbc_news_embeddings`,
ml_generate_embedding_result',
(
SELECT
ml_generate_embedding_result,
content AS title
FROM
ML.GENERATE_EMBEDDING(
MODEL`toolbox-starfox.vector_search_demo.embeddings_model_demo`,
(
SELECT "PlayStation 3 chip to be unveiled" AS content
),
STRUCT(
TRUE AS flatten_json_output
)
)
),
distance_type => 'COSINE'
);

The result is significant: we can see that Sony PSP, XboX 2 and Sony are identified as words semantically similar to PlayStation, which means that the vector search has worked well. Of course, we can always improve the result by optimizing the model options 👇.
ℹ️ You can usenum_lists
with the argumentfractions_list_to_search
functionVECTOR_SEARCH
to optimize vector search.
- If your data is divided into many small groups in vector space :
- Use a high value for
num_lists
- Use a low value for
fractions_list_to_search
- Use a high value for
- If your data is divided into a few large groups :
- Use a low value for
num_lists
- Use a high value for
fractions_list_to_search
- Use a low value for
If you do not specify a value for num_lists
BigQuery will automatically calculate one.
The integration of vector search into BigQuery represents a significant step forward for businesses. This feature is particularly advantageous for SMEs, giving them access to advanced analysis capabilities. It's more than just a technical enhancement; it represents a real transformation lever for companies, enabling them to fully exploit the potential of their data in an increasingly digital and competitive world.

A need, a question?
Write to us at hello@starfox-analytics.com.
Our team will get back to you as soon as possible.